Synthetic intelligence has grown past language-focused methods, evolving into fashions able to processing a number of enter varieties, comparable to textual content, photos, audio, and video. This space, generally known as multimodal studying, goals to copy the pure human capacity to combine and interpret various sensory knowledge. Not like standard AI fashions that deal with a single modality, multimodal generalists are designed to course of and reply throughout codecs. The objective is to maneuver nearer to creating methods that mimic human cognition by seamlessly combining various kinds of data and notion.
The problem confronted on this discipline lies in enabling these multimodal methods to display true generalization. Whereas many fashions can course of a number of inputs, they usually fail to switch studying throughout duties or modalities. This absence of cross-task enhancement—generally known as synergy—hinders progress towards extra clever and adaptive methods. A mannequin could excel in picture classification and textual content technology individually, however it can’t be thought of a strong generalist with out the power to attach expertise from each domains. Reaching this synergy is important for creating extra succesful, autonomous AI methods.
Many present instruments rely closely on massive language fashions (LLMs) at their core. These LLMs are sometimes supplemented with exterior, specialised elements tailor-made to picture recognition or speech evaluation duties. For instance, current fashions comparable to CLIP or Flamingo combine language with imaginative and prescient however don’t deeply join the 2. As a substitute of functioning as a unified system, they rely upon loosely coupled modules that mimic multimodal intelligence. This fragmented method means the fashions lack the inner structure needed for significant cross-modal studying, leading to remoted job efficiency fairly than holistic understanding.
Researchers from the Nationwide College of Singapore (NUS), Nanyang Technological College (NTU), Zhejiang College (ZJU), Peking College (PKU), and others proposed an AI framework named Common-Degree and a benchmark referred to as Common-Bench. These instruments are constructed to measure and promote synergy throughout modalities and duties. Common-Degree establishes 5 ranges of classification based mostly on how nicely a mannequin integrates comprehension, technology, and language duties. The benchmark is supported by Common-Bench, a big dataset encompassing over 700 duties and 325,800 annotated examples drawn from textual content, photos, audio, video, and 3D knowledge.
The analysis methodology inside Common-Degree is constructed on the idea of synergy. Fashions are assessed by job efficiency and their capacity to exceed state-of-the-art (SoTA) specialist scores utilizing shared data. The researchers outline three forms of synergy—task-to-task, comprehension-generation, and modality-modality—and require rising functionality at every stage. For instance, a Degree-2 mannequin helps many modalities and duties, whereas a Degree-4 mannequin should exhibit synergy between comprehension and technology. Scores are weighted to scale back bias from modality dominance and encourage fashions to help a balanced vary of duties.
The researchers examined 172 massive fashions, together with over 100 top-performing MLLMs, in opposition to Common-Bench. Outcomes revealed that the majority fashions don’t display the wanted synergy to qualify as higher-level generalists. Even superior fashions like GPT-4V and GPT-4o didn’t attain Degree 5, which requires fashions to make use of non-language inputs to enhance language understanding. The very best-performing fashions managed solely fundamental multimodal interactions, and none confirmed proof of whole synergy throughout duties and modalities. As an example, the benchmark confirmed 702 duties assessed throughout 145 expertise, but no mannequin achieved dominance in all areas. Common-Bench’s protection throughout 29 disciplines, utilizing 58 analysis metrics, set a brand new customary for comprehensiveness.
This analysis clarifies the hole between present multimodal methods and the best generalist mannequin. The researchers deal with a core challenge in multimodal AI by introducing instruments prioritizing integration over specialization. With Common-Degree and Common-Bench, they provide a rigorous path ahead for assessing and constructing fashions that deal with varied inputs and study and purpose throughout them. Their method helps steer the sphere towards extra clever methods with real-world flexibility and cross-modal understanding.
Try the Paper and Venture Web page. All credit score for this analysis goes to the researchers of this undertaking. Additionally, be at liberty to comply with us on Twitter and don’t overlook to affix our 90k+ ML SubReddit.
Right here’s a short overview of what we’re constructing at Marktechpost:
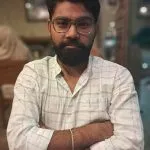
Nikhil is an intern marketing consultant at Marktechpost. He’s pursuing an built-in twin diploma in Supplies on the Indian Institute of Expertise, Kharagpur. Nikhil is an AI/ML fanatic who’s at all times researching purposes in fields like biomaterials and biomedical science. With a robust background in Materials Science, he’s exploring new developments and creating alternatives to contribute.