Multimodal modeling focuses on constructing methods to know and generate content material throughout visible and textual codecs. These fashions are designed to interpret visible scenes and produce new photographs utilizing pure language prompts. With rising curiosity in bridging imaginative and prescient and language, researchers are working towards integrating picture recognition and picture era capabilities right into a unified system. This method eliminates the necessity for separate pipelines and opens the trail to extra coherent and clever interactions throughout modalities.
A key problem on this area is to develop architectures that deal with each understanding and era with out compromising the standard of both. Fashions want to understand complicated visible ideas and produce high-quality photographs matching consumer prompts. The problem lies in figuring out appropriate image representations and coaching procedures that help each duties. This downside turns into extra evident when the identical mannequin is anticipated to interpret detailed textual content descriptions and generate visually correct outputs based mostly on them. It requires alignment of semantic understanding and pixel-level synthesis.
Earlier approaches have usually used Variational Autoencoders (VAEs) or CLIP-based encoders to signify photographs. VAEs are environment friendly for reconstruction however encode lower-level options, typically resulting in much less informative representations. CLIP-based encoders present high-level semantic embeddings by studying from large-scale image-text pairs. Nonetheless, CLIP was not constructed for picture reconstruction, making it difficult to make use of for era except paired with fashions like diffusion decoders. By way of coaching, Imply Squared Error (MSE) is broadly used for simplicity however tends to provide deterministic outputs. To enhance era range and high quality, researchers have turned to Movement Matching, which introduces managed stochasticity and higher fashions the continual nature of picture options.
Researchers from Salesforce Analysis, in collaboration with the College of Maryland and a number of other tutorial establishments, launched BLIP3-o, a household of unified multimodal fashions. The mannequin adopts a dual-stage coaching technique the place picture understanding is realized first, adopted by picture era. The proposed system leverages CLIP embeddings to signify photographs and integrates them with a diffusion transformer to synthesize new visible outputs. In contrast to earlier joint coaching strategies, the sequential method maintains the power of every activity independently. The diffusion module is skilled whereas protecting the autoregressive spine frozen, avoiding activity interference. To enhance alignment and visible constancy, the workforce additionally curated BLIP3o-60k, a high-quality instruction-tuning dataset created by prompting GPT-4o throughout diversified visible classes, together with scenes, objects, gestures, and textual content. They developed two mannequin variations: an 8-billion parameter mannequin skilled with proprietary and public information, and a 4-billion model utilizing solely open-source information.
The picture era pipeline of BLIP3-o is constructed on Qwen2.5-VL giant language fashions. Prompts are processed to provide visible options refined via a Movement Matching diffusion transformer. This transformer relies on the Lumina-Subsequent structure, optimized for pace and high quality with 3D rotary place embedding and grouped-query consideration. The mannequin encodes every picture into 64 fixed-length semantic vectors, no matter decision, which helps compact storage and environment friendly decoding. The analysis workforce used a large-scale dataset of 25 million photographs from sources like CC12M, SA-1B, and JourneyDB to coach the fashions. They prolonged it with 30 million proprietary samples for the 8B mannequin. In addition they included 60k instruction-tuning samples masking difficult prompts reminiscent of complicated gestures and landmarks, generated through GPT-4o.
By way of efficiency, BLIP3-o demonstrated prime scores throughout a number of benchmarks. The 8B mannequin achieved a GenEval rating of 0.84 for picture era alignment and a WISE rating of 0.62 for reasoning skill. Picture understanding scored 1682.6 on MME-Notion, 647.1 on MME-Cognition, 50.6 on MMMU, and 83.1 on each VQAv2 and TextVQA datasets. A human analysis evaluating BLIP3-o 8B with Janus Professional 7B confirmed that BLIP3-o was most well-liked 50.4% of the time for visible high quality and 51.5% for immediate alignment. These outcomes are supported by statistically important p-values (5.05e-06 and 1.16e-05), indicating the prevalence of BLIP3-o in subjective high quality assessments.
This analysis outlines a transparent answer to the twin problem of picture understanding and era. CLIP embeddings, Movement Matching, and a sequential coaching technique show how the issue might be approached methodically. The BLIP3-o mannequin delivers state-of-the-art outcomes and introduces an environment friendly and open method to unified multimodal modeling.
Try the Paper, GitHub Web page and Mannequin on Hugging Face. All credit score for this analysis goes to the researchers of this undertaking. Additionally, be at liberty to observe us on Twitter and don’t neglect to hitch our 90k+ ML SubReddit.
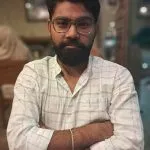
Nikhil is an intern guide at Marktechpost. He’s pursuing an built-in twin diploma in Supplies on the Indian Institute of Expertise, Kharagpur. Nikhil is an AI/ML fanatic who’s at all times researching purposes in fields like biomaterials and biomedical science. With a powerful background in Materials Science, he’s exploring new developments and creating alternatives to contribute.
